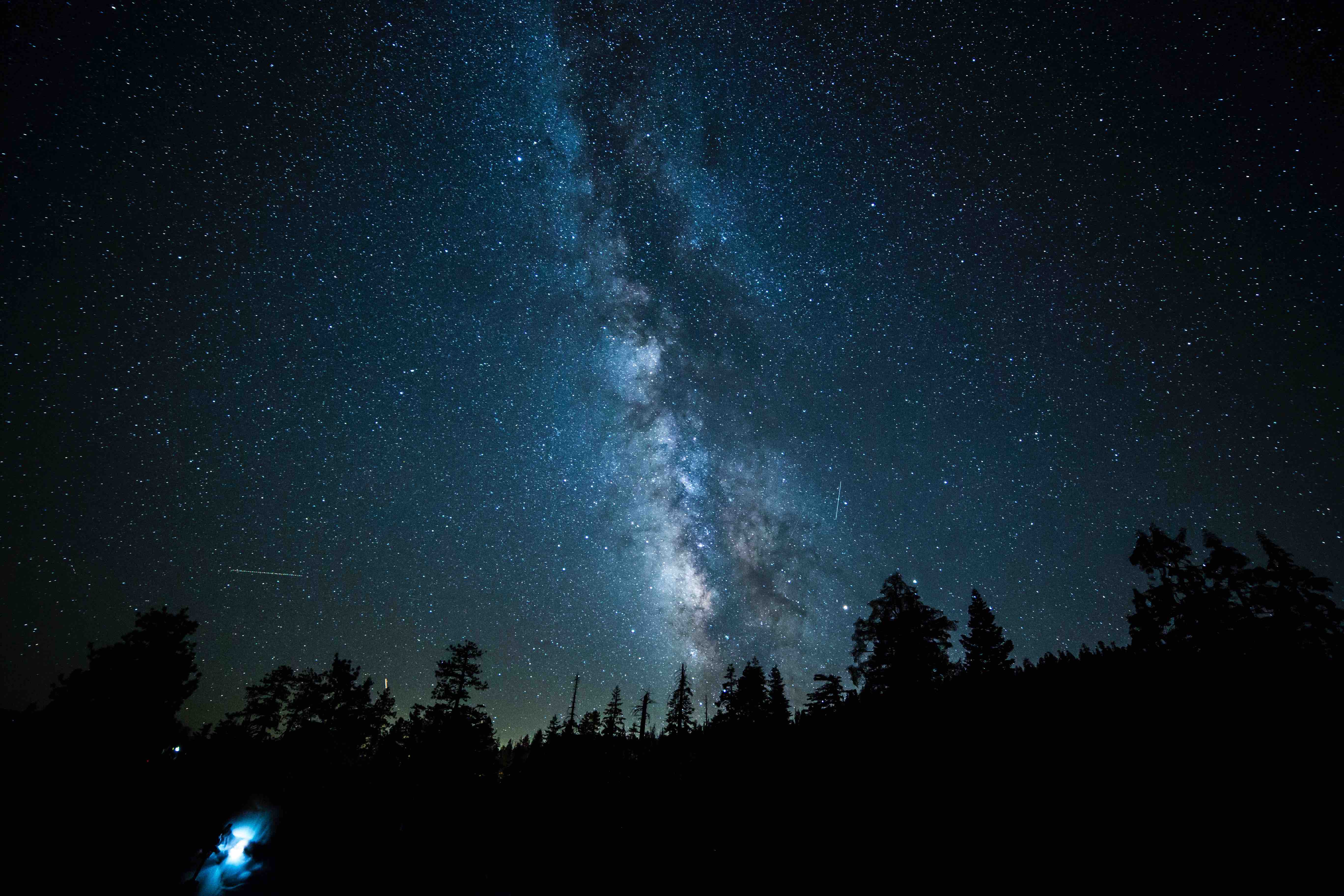
I am Eeshan, a graduate student pursuing M.Sc. (Informatique) at Mila, Université de Montréal. I graduated with B.Tech. in Electrical Engineering with Second Major in Computer Science and Engineering from Indian Institute of Technology, Kanpur.
I am interested in Machine Learning and its applications for Learning Better Representations, especially for Computer Vision. I am also interested in Reinforcement Learning and Probabilistic Modeling.
Apart from this, I enjoy Mathematics, Astronomy, and Philosophy. Also, I am a self-taught Indian Bamboo Flute player.